Real-time Holistic Robot Pose Estimation with Unknown States
-
Shikun Ban -
Juling Fan - Wentao Zhu
- Xiaoxuan Ma
- Yu Qiao
- Yizhou Wang
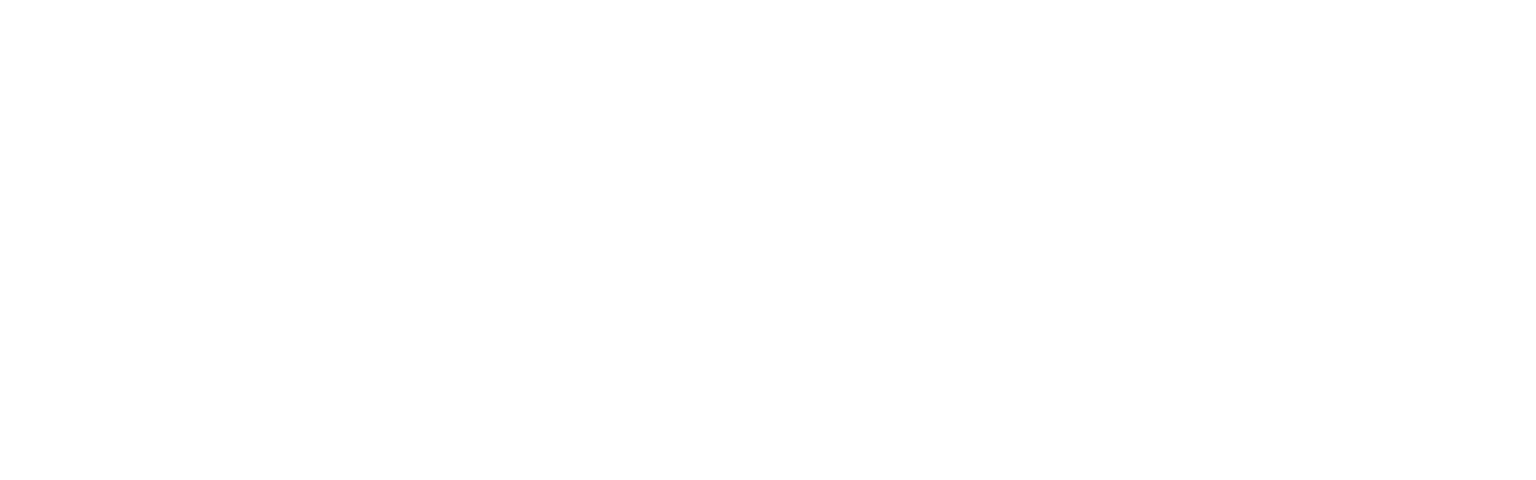
Abstract
Estimating robot pose from RGB images is a crucial problem in computer vision and robotics. While previous methods have achieved promising performance, most of them presume full knowledge of robot internal states, e.g. ground-truth robot joint angles. However, this assumption is not always valid in practical situations. In real-world applications such as multi-robot collaboration or human-robot interaction, the robot joint states might not be shared or could be unreliable. On the other hand, existing approaches that estimate robot pose without joint state priors suffer from heavy computation burdens and thus cannot support real-time applications.
This work introduces an efficient framework for real-time robot pose estimation from RGB images without requiring known robot states. Our method estimates camera-to-robot rotation, robot state parameters, keypoint locations, and root depth, employing a neural network module for each task to facilitate learning and sim-to-real transfer. Notably, it achieves inference in a single feedforward pass without iterative optimization. Our approach offers a 12-time speed increase with state-of-the-art accuracy, enabling real-time holistic robot pose estimation for the first time.
Framework
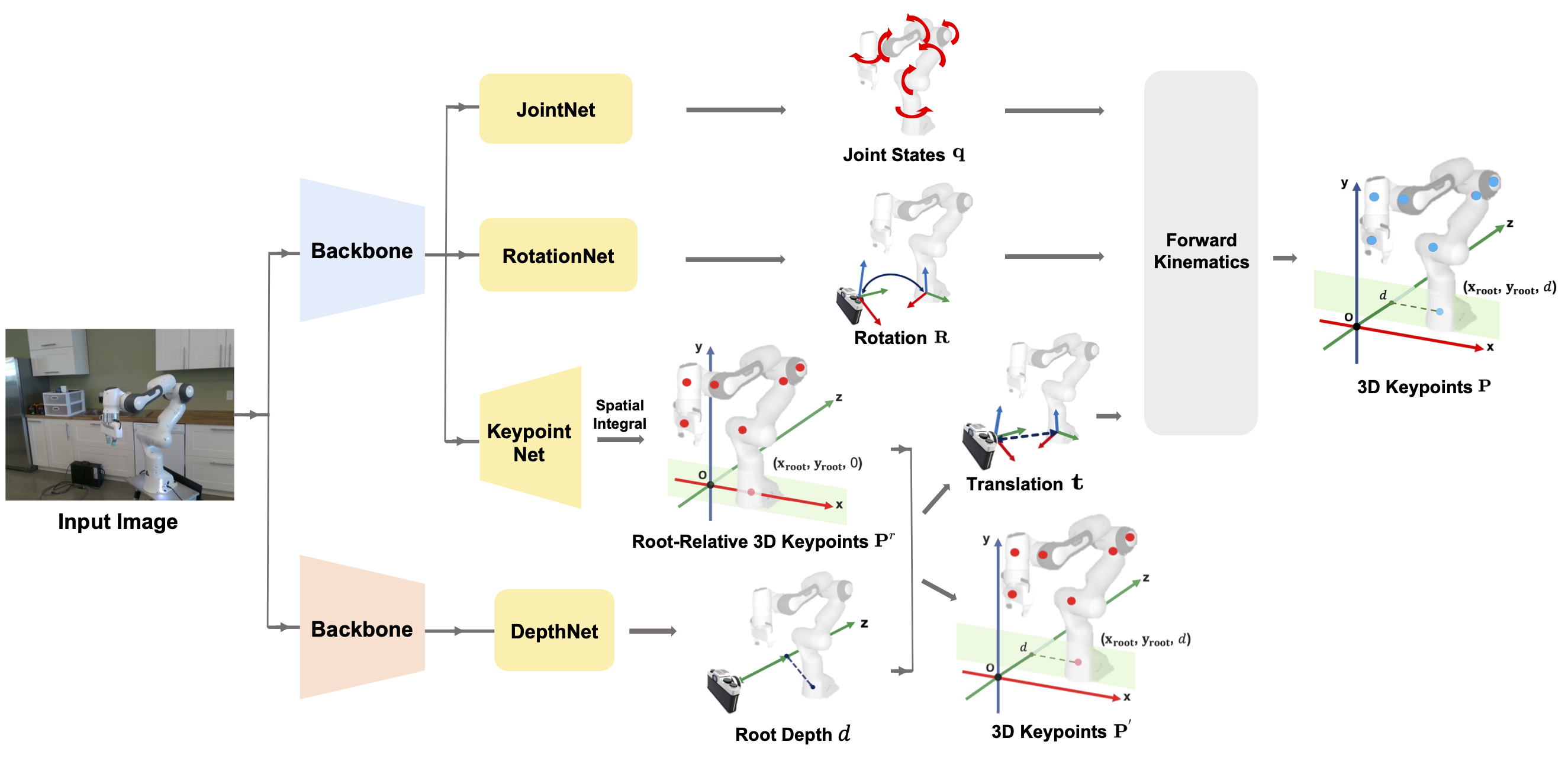
We factorize the holistic robot pose estimation task into several sub-tasks, including the estimation of camera-to-robot rotation, robot joint states, root depth, and root-relative keypoint locations. We then design corresponding neural network modules for each sub-task.
Results
Qualitative Comparison on DREAM dataset
Input RoboPose Ours
Qualitative comparison presented above illustrates our approach's ability to produce more stable and accurate estimates in multiple videos from the real-world Panda Datasets.
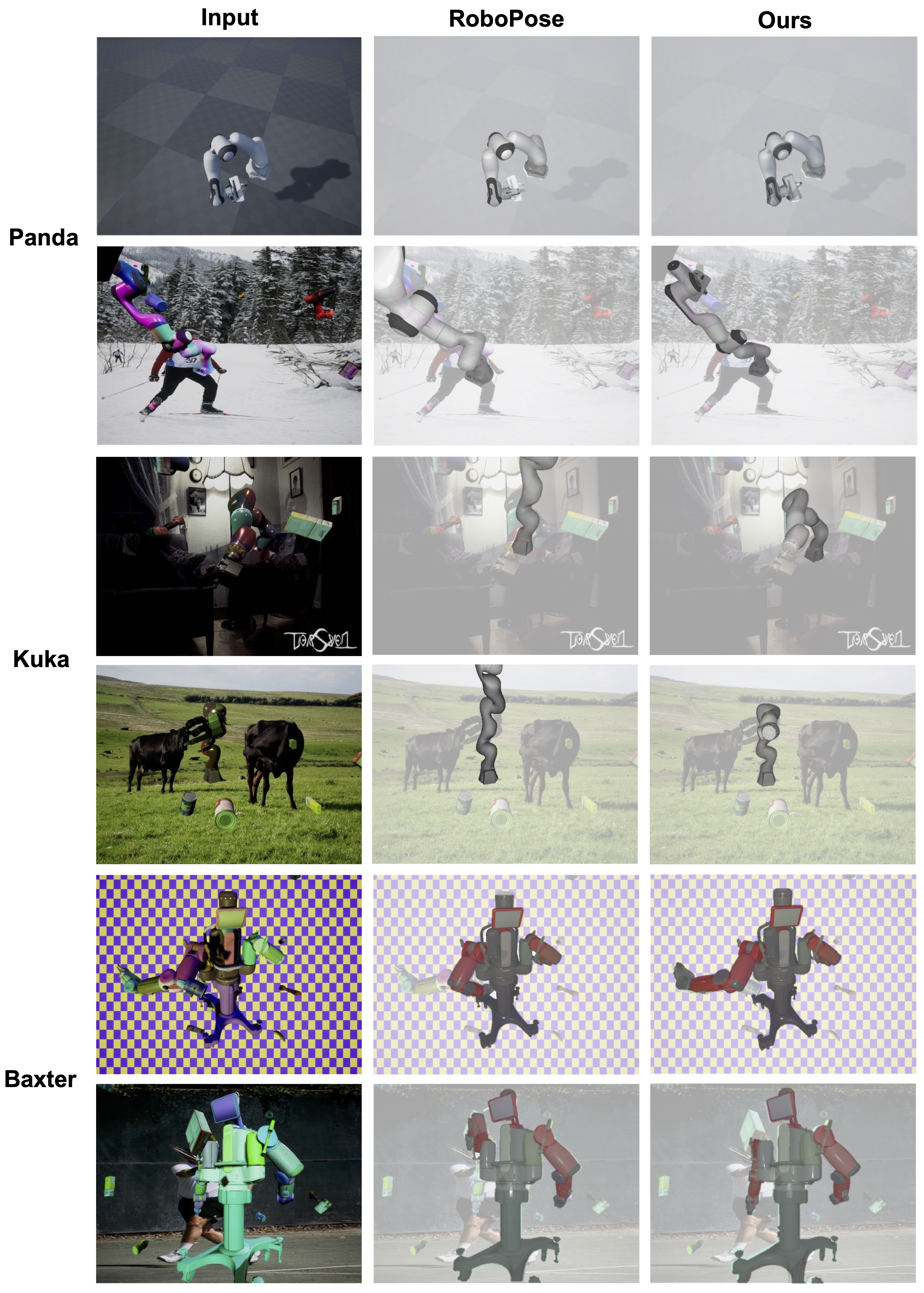
Qualitative comparison on images of different robots is presented above. It illustrates that our approach's ability to produce high-quality estimates across different robot morphologies. Particularly, our approach demonstrates superior performance in highly challenging cases, including self-occlusions, truncations, and extreme lighting conditions.
Qualitative Comparison on In-the-wild Images
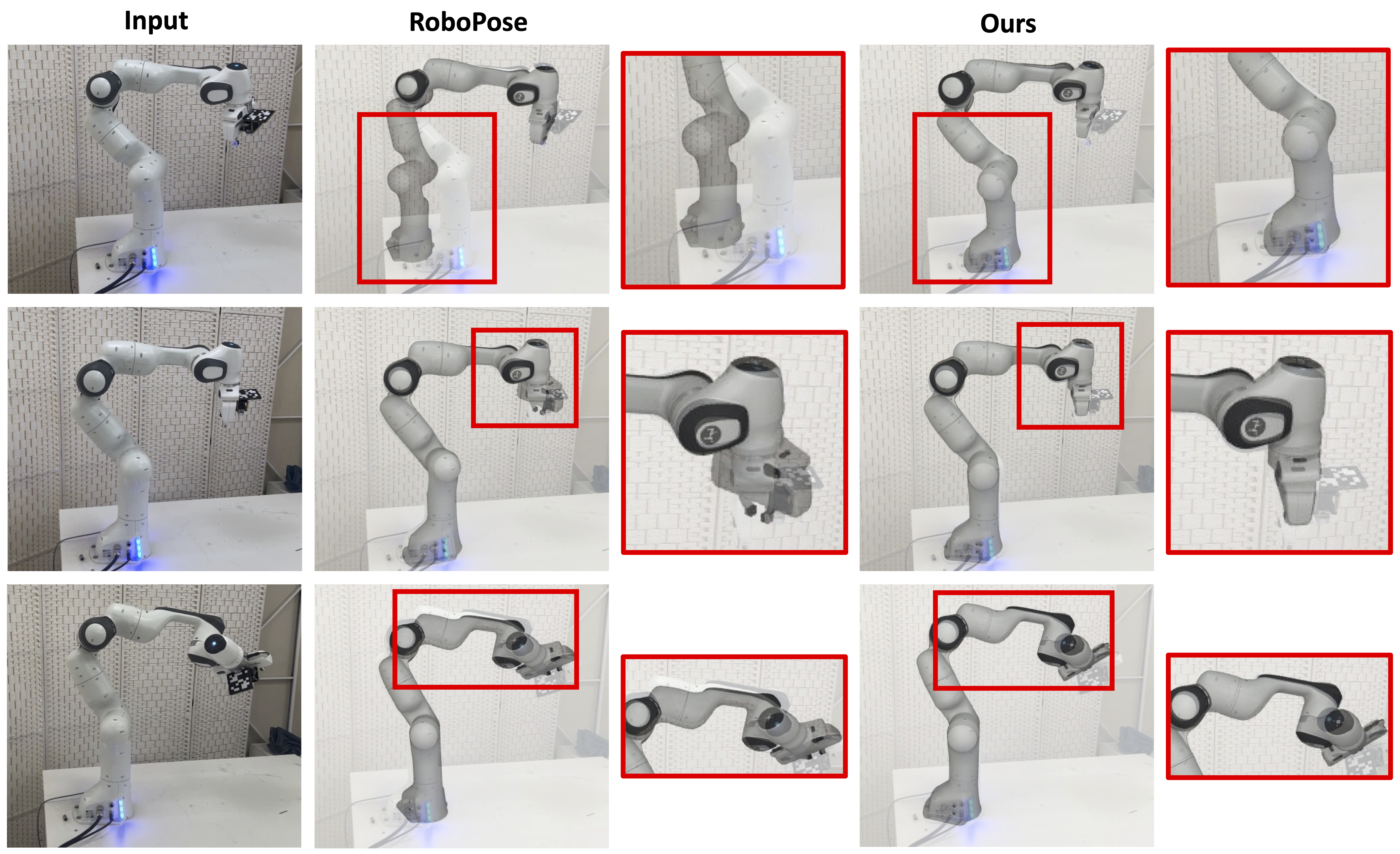
Qualitative results for in-the-wild laboratory images are presented above. No markers were used in the process. For instance, in the image located in the upper row, our method demonstrates a more accurate estimation of the pose of the robot's base.
Citation
@article{ban2024real,
title={Real-time Holistic Robot Pose Estimation with Unknown States},
author={Ban, Shikun and Fan, Juling and Zhu, Wentao and Ma, Xiaoxuan and Qiao, Yu and Wang, Yizhou},
journal={arXiv preprint arXiv:2402.05655},
year={2024}
}
Template courtesy of Jon Barron.